Plenary Talks
Title: Theory and Examples of One-Bit Secret Sharing
Hiroki Koga, University of Tsukuba
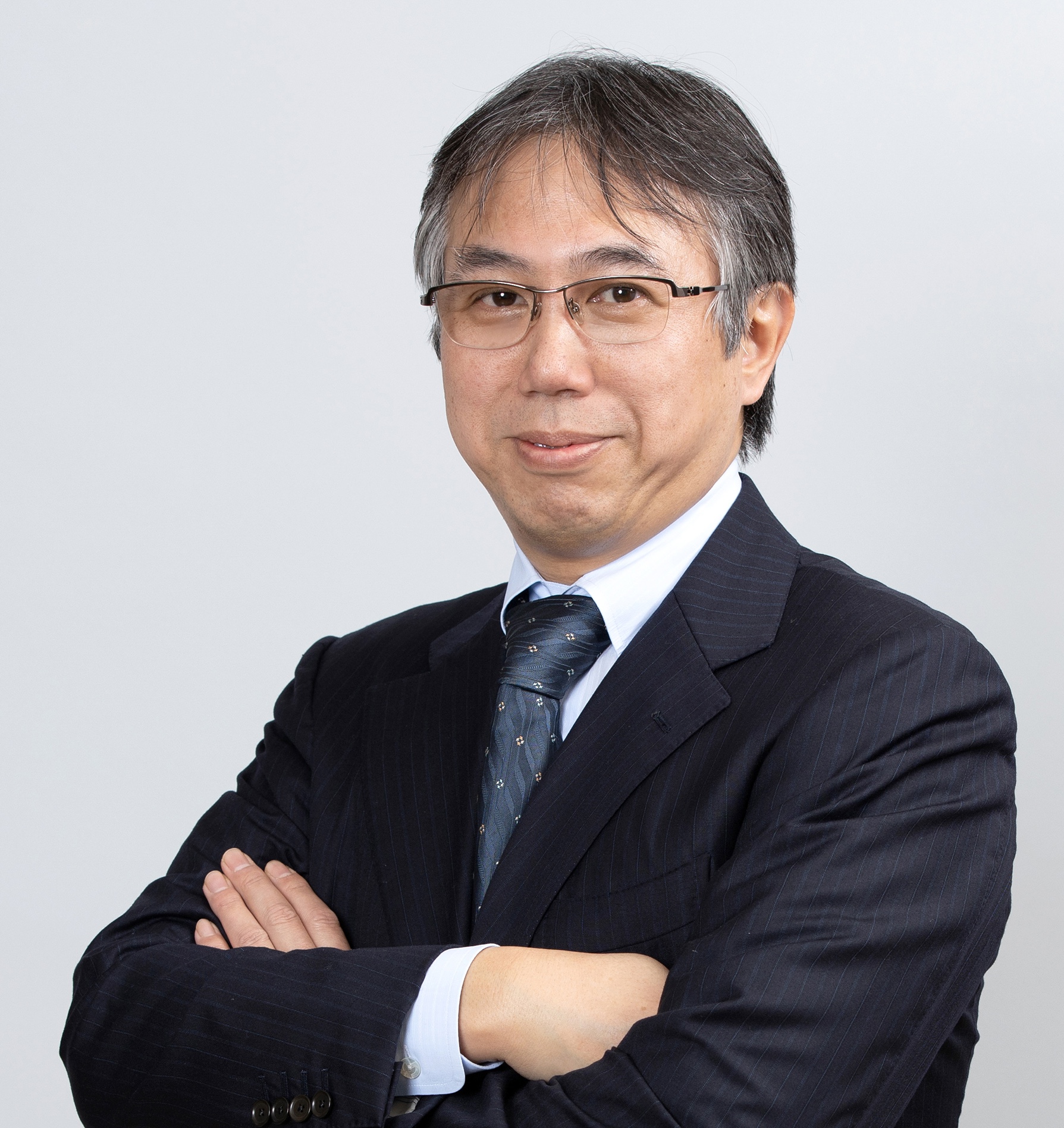
Secret sharing is one of the important cryptographic primitives. In a secret sharing scheme, a dealer generates n shares from a secret S and distributes the n shares to n respective participants. If we consider the t-out-of-n threshold access structure, S is correctly recovered from arbitrary more than or equal to t shares, while no information on S is revealed from less than t shares. It is well-known that Shamir's scheme using a random polynomial of order at most t-1 gives a secret sharing scheme realizing the t-out-of-n threshold access structure. In Shamir's scheme, a secret takes values in the same alphabet as the n shares.
In my talk, we focus on nonconventional secret sharing schemes for the case where a secret S is one-bit. Visual cryptography schemes that originate from Naor and Shamir's paper in 1994 can be regarded as one-bit secret sharing schemes. Recently, Bogdanov, Guo and Komargodski gave a new lower bound of the share size by using a new theory for the one-bit secret sharing.
We first give interesting examples of the one-bit secret sharing schemes. Next, we give a construction of the one-bit secret sharing scheme realizing the t-out-of-p access structure, where p is an arbitrary prime number. In this scheme we actually construct a function with p variables that corresponds to a multiple of the difference between the conditional probability of the shares given S=0 and S=1. Surprisingly, the discrete Fourier transform of this function takes only two values, 0 and 1. We use this property to guarantee the security of the one-bit secret S against any t-1 shares. We also discuss an extension of this scheme to the case of a multi-valued secret and a relationship of this scheme to the orthogonal array.
Hiroki Koga received B.E., M.E. and D.E. degrees from University of Tokyo, in 1990, 1992 and 1995, respectively. From 1995 to 1999, he was a Research Associate in Graduate School of Engineering, University of Tokyo. Since 1999, he has been with University of Tsukuba, where he is currently a Professor of Institute of Systems and Information. His research interests include Shannon theory and information security. Dr. Koga is a member of IEICE,and IEEE.
Title: A Journey in Information Theory: From Second-Order Rates to Common Information
Vincent Y. F. Tan, National University of Singapore

In this talk, I will discuss my journey in information theory. The story starts from my initial interest in establishing second-order coding rates for various network information theory problems including the Slepian--Wolf distributed lossless source coding problem and various (simple) variants of multiple access and interference channels. These led to my interest in using Rényi resolvability to establish second-order rates in problems involving equivocations such as the wiretap channel. The above studies eventually inspired me to the study of various common information quantities and the resolution of some open problems therein.
Vincent Y. F. Tan (S'07-M'11-SM'15) was born in Singapore in 1981. He received the B.A. and M.Eng. degrees in electrical and information science from Cambridge University in 2005, and the Ph.D. degree in electrical engineering and computer science (EECS) from the Massachusetts Institute of Technology (MIT) in 2011. He is currently a Professor with the Department of Mathematics and the Department of Electrical and Computer Engineering (ECE), National University of Singapore (NUS). His research interests include information theory, machine learning, and statistical signal processing.
Dr. Tan is an elected member of the IEEE Information Theory Society Board of Governors. He was an IEEE Information Theory Society Distinguished Lecturer from 2018 to 2019. He received the MIT EECS Jin-Au Kong Outstanding Doctoral Thesis Prize in 2011, the NUS Young Investigator Award in 2014, the Singapore National Research Foundation (NRF) Fellowship (Class of 2018), the Engineering Young Researcher Award in 2018, and the NUS Young Researcher Award in 2019. A dedicated educator, he was awarded the Engineering Educator Award in 2020 and 2021 and the (university level) Annual Teaching Excellence Award in 2022. He is currently serving as a Senior Area Editor for the IEEE Transactions on Signal Processing and as an Associate Editor in Machine Learning and Statistics for the IEEE Transactions on Information Theory. He also regularly serves as an Area Chair of prominent machine learning conferences such as the International Conference on Learning Representations (ICLR) and the Conference on Neural Information Processing Systems (NeurIPS).
Title: A Journey in belief propagation: From practical LDPC decoders to near-maximum likelihood Reed-Solomon decoders
Yeong-Luh Ueng, National Tsing Hua University

In this talk, I will present an overview of several results that I have gradually developed over the last decade for message passing scheduling in belief propagation (BP) decoders for both low-density and high-density parity-check codes. Low-density parity-check (LDPC) codes, because of BP decoding, have become one of the most popular coding schemes for both communication and storage systems. Hardware implementation widely employs several fixed scheduling techniques, including layered and shuffled scheduling, to enhance convergence rate and error-rate performance. Although BP is very successful in LDPC codes, it performs poorly when applied to high-density parity-check codes like Reed-Solomon (RS). Recently, we applied the Nested-Polling Residual Belief Propagation (NP-RBP) method to the high-density Tanner graph of RS codes. The NP-RBP decoding approach, like the popular adaptive BP (ABP) decoding approach, involves adaptation in a binary parity-check matrix based on the reliability of variable nodes, and is able to provide an error rate performance close to the maximum-likelihood (ML) bound for short codes. For long codes, we use a bit-flipping (BF) technique to correct a subset of errors in the most reliable variable nodes, thereby improving error-rate performance. When the proposed NP-RBP-BF decoding is applied to the (255, 239) RS code, it can provide a gain of about 0.4 dB compared to the ABP decoding and the performance gap to the ML bound can be narrowed to about 0.25 dB at a frame error rate of 2 × 10^{-3}.
Yeong-Luh Ueng (M’05-SM’15) received his Ph.D. degree in communication engineering from the National Taiwan University, Taipei, Taiwan, in 2001. From 2001 to 2005, he was with a private communication technology company, where he focused on the design and development of various wireless chips. Since December 2005, he has been a member of the faculty at the National Tsing Hua University, Hsinchu, Taiwan, where he is currently a Full Professor with the Department of Electrical Engineering and the Institute of Communications Engineering. In 2016, he was the recipient of the Wu Ta-You Memorial Award from the Ministry of Science and Technology (MOST). In 2018, he was the recipient of the Outstanding Electrical Engineering Professor award from the Chinese Electrical Engineering Association, as well as the Outstanding Research Award from the Ministry of Science and Technology (MOST), Taiwan.
Dr. Ueng is currently an Associate Editor for IEEE Signal Processing Letters and Vice- Chair of IEEE Communications Society Taipei Chapter. In the past, he has also served as a guest editor for IEEE Journal on Emerging and Selected Topics in Circuits and Systems (2020). He was also Chair of IEEE Information Theory Society Taipei Chapter (2019-2021). He served as Co-Chair of 2018 IEEE Global Conference on Signal and Information Processing, Symposium on Design, Implementation and Optimization of Deep Learning for Wireless Communications, Invited Session Organizer of 2017 IEEE Information Theory Workshop (ITW), and Tutorial Chair of 2019 IEEE Workshop on Signal Processing Systems (SiPS) and 2023 IEEE International Symposium on Information Theory. His research interests include coding theory, wireless communications, communication ICs, and deep learning.